Emotions in politics
Emotions are everywhere. Especially in politics.
Recently, The Oxford Dictionaries announced that its Word of the Year 2016 is post-truth.
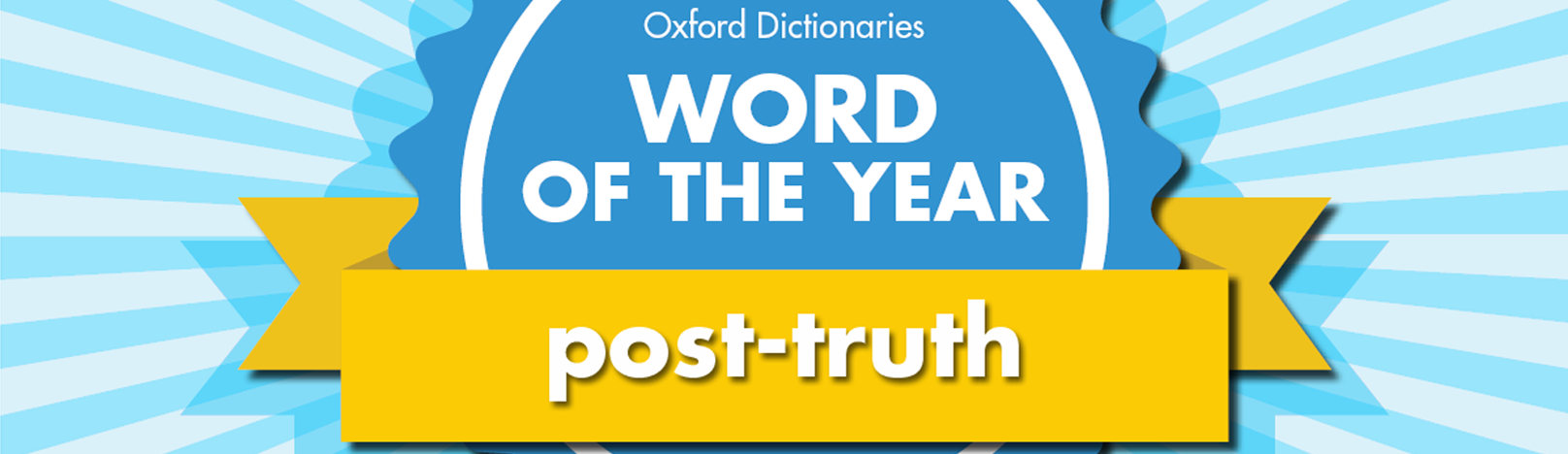
‘relating to or denoting circumstances in which objective facts are less influential
in shaping public opinion than appeals to emotion and personal belief’
- 'Post-truth' according to The Oxford Dictionaries
The frequent use of a word such as 'post-truth' indicates that people seem to think that politics are increasingly prefering emotions to fact. Pathos to logos. But is this true? We investigated how emotions are showing in the Danish parliament and on Danish politicians' Twitter feeds. The Danish parliament dataset was extracted using web scraping from transcipted parliament meetings and the twitter dataset was generated using the twitter API and contains tweets from 149 Danish politicians.
Emotions over time
Let's get an overview of the emotional spectrum by looking at the weekly average of emotions on Danish politicians' Twitter and in the parliament:
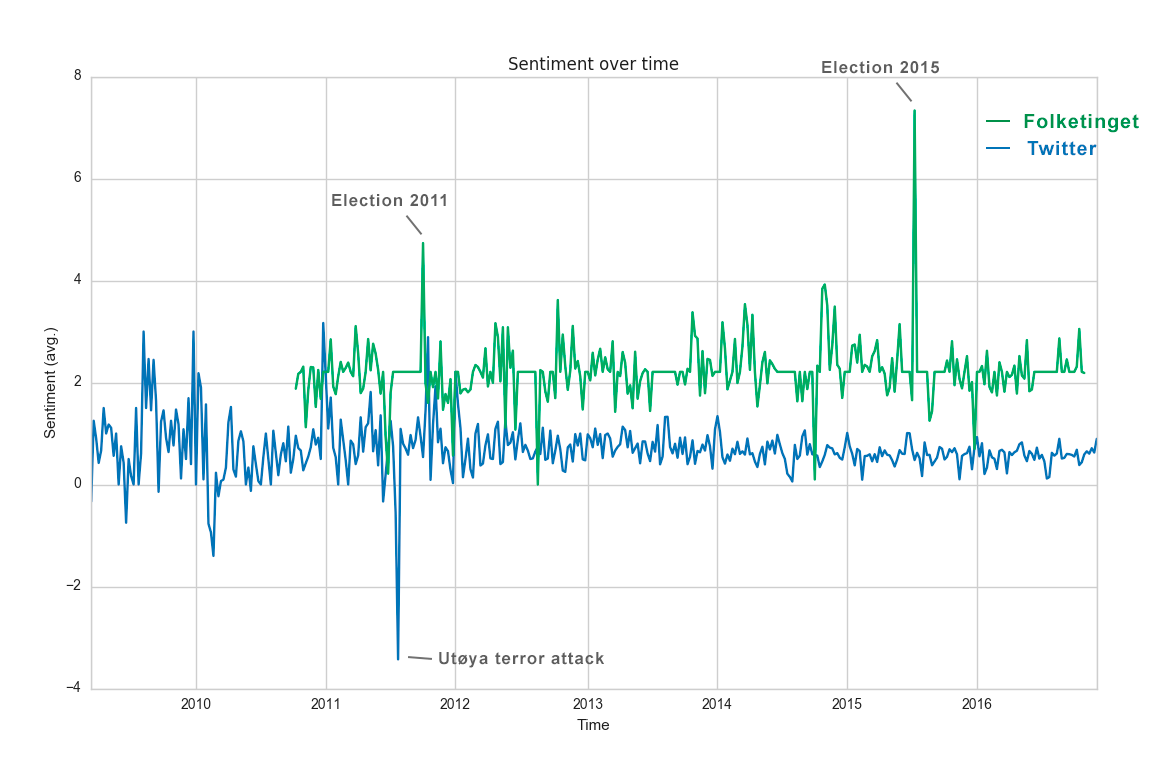
The graphs seem to be quite well correlated. It should be noted that the method of calculating the sentiments was to add up positive and negative sentiment values of each word in a text. Averaging the blocks of text makes it more likely for longer text blocks to have extreme values (positive or negative), which is probably why the sentiment of the parliament is generally higher than on twitter.
How are politicians sentiment in the parliament compared to their twitter sentiment?
We sometimes experience that people behave different in real life compared to their online behaviour. Examining the sentiment of tweets from danish politicians and comparing it to the sentiment of when they are talking in the parliament meetings shows the average and standard deviation of each politicians sentiment both on twitter and in the meetings. A high average sentiment and low standard deviation sentiment implies a high sentiment in general while a high standard deviation implies a high level of expressivenes.
Average Sentiment
Standard Deviation Sentiment
The chart suggests that Henrik Sass Larsen has a very high sentiment on Twitter while having a more average sentiment in the parliament. Furthermore Pernille Rosenkrantz has the highest standard deviation on twitter, this implies that she is very expressive in both negative and positive tweets.
How are emotions showing on Danish politicians' Twitter feeds?
The sentiment averages of politicians own tweets (retweets not counted) was used as a basis for creating lists of top most positive tweets and top most negative tweets.
Top 3 positive tweets:
-
I dag er det DFs fødselsdag hurra hurra hurra Vi sikkert mange gaver får Som vi har ønsket os i år For 21 år er vel en gave værd #dkpol
-
"Messerschmidt har aldrig sat sig ind i, hvordan EU fungerer..." (citat Anna Rosbach i Berlingske). Ha-ha-ha-ha-ha-ha-ha-ha-ha-ha..! #dkpol
-
Hej @FradragNu - Det er mig der takker for super god inspiration til iværksætterpolitik hos @Spolitik - held og lykke med fradrag.nu #dkpol
DF seems to be the happiest party on Twitter, but...
Søren Espersen's tweet that is ranked as the second happiest in our records is a great example of a quirk when calculating sentiments using a list of labelled words.
When reading the tweet as a human, it is obvious that the tweet is sarcastic and not very positive. However, being limited to look at each word, our algorithm count the long string of Ha-ha-ha... as very positive.
Now, let's have a look at the top 3 negative tweets:
-
Fuck fuck fuck Andrew er ikke videre. Øv!!! En voksen mand m rock under vesten - og så kunne han synge. Fuck! #xfactordr
-
WTF? @sorenpind kobler skærpet straf for voldtægt og skærpet straf for falsk anklage #usagligt #sexisme #dkpol https://t.co/eNbeEtoH5x
-
@ElisabethMJ @Kvindemuseet @finanslov øv, øv, øv, øv og sgu altså. Helt forkert. @rasmusnordqvist har du set det her?
The most negative tweets ranges from angry, to sad, to ... X-Factor.
One interesting observation is the distribution of positive/negative politicians based on their party. The top positive tweets mostly include right-wing politicians, while the top negative tweets only include left-wing politicians.
-
I dag er det DFs fødselsdag hurra hurra hurra Vi sikkert mange gaver får Som vi har ønsket os i år For 21 år er vel en gave værd #dkpol
-
"Messerschmidt har aldrig sat sig ind i, hvordan EU fungerer..." (citat Anna Rosbach i Berlingske). Ha-ha-ha-ha-ha-ha-ha-ha-ha-ha..! #dkpol
-
Hej @FradragNu - Det er mig der takker for super god inspiration til iværksætterpolitik hos @Spolitik - held og lykke med fradrag.nu #dkpol
-
@KlausKblog Fantastisk! Super godt gået!! Jeg bliver glad i låget over folk som os.. haha :-)
-
Jeg var enig med HTS i denne del: "Danmark længe leve! Hurra, hurra, hurra!" #ftlive #dkpol
-
Fuck fuck fuck Andrew er ikke videre. Øv!!! En voksen mand m rock under vesten - og så kunne han synge. Fuck! #xfactordr
-
WTF? @sorenpind kobler skærpet straf for voldtægt og skærpet straf for falsk anklage #usagligt #sexisme #dkpol https://t.co/eNbeEtoH5x
-
@ElisabethMJ @Kvindemuseet @finanslov øv, øv, øv, øv og sgu altså. Helt forkert. @rasmusnordqvist har du set det her?
-
Frygtelige nyheder fra Paris- igen. Al den vold, terror og drab på uskyldige mennesker. Tragisk.
-
Voldtægt er aldrig din skyld. Offer skal ikke efterlades med skam og angst for ikke at blive taget alvorligt #dkpol https://t.co/9OgTSdqe08